PROJECT SUMMARY

Quantifying and Measuring Bias and Engagement
Focus Area(s): News & Media, Health
Research Program: Machines, Data
Automated decision making systems and machines – including search engines, intelligent assistants, and recommender systems – are designed, evaluated, and optimised by defining frameworks that model the users who are going to interact with them. These models are typically a simplified representation of users (e.g., using the relevance of items delivered to the user as a surrogate for system quality) to operationalise the development process of such systems. A grand open challenge is to make these frameworks more complete, by including new aspects such as fairness, that are as important as the traditional definitions of quality, to inform the design, evaluation and optimisation of such systems.
Recent developments in machine learning and information access communities attempt to define fairness-aware metrics to incorporate into these frameworks. However, there are a number of research questions related to quantifying and measuring bias and engagement that remain unexplored:
- Is it possible to measure bias by observing users interacting with search engines, recommender systems, or intelligent assistants?
- How do users perceive fairness, bias and trust? How can these perceptions be measured effectively?
- To what extent can sensors in wearable devices and interaction logging (e.g., CTR, app swipes, notification dismissal, etc) inform the measurement of bias and engagement?
- Are the implicit signals captured from sensors and interaction logs correlated with explicit human ratings w.r.t. bias and engagement?
The research aims to address the research questions above by focusing on information access systems that involve automated decision-making components. This is the case for search engines, intelligent assistants, and recommender systems. The methodologies considered to address these questions include lab user studies (e.g., Wizard of Oz experiments with intelligent assistants), and the use of crowdsourcing platforms (e.g., Amazon Mechanical Turk). The data collection processes include: logging human-system interactions; sensor data collected using wearable devices; and questionnaires.
RESEARCHERS
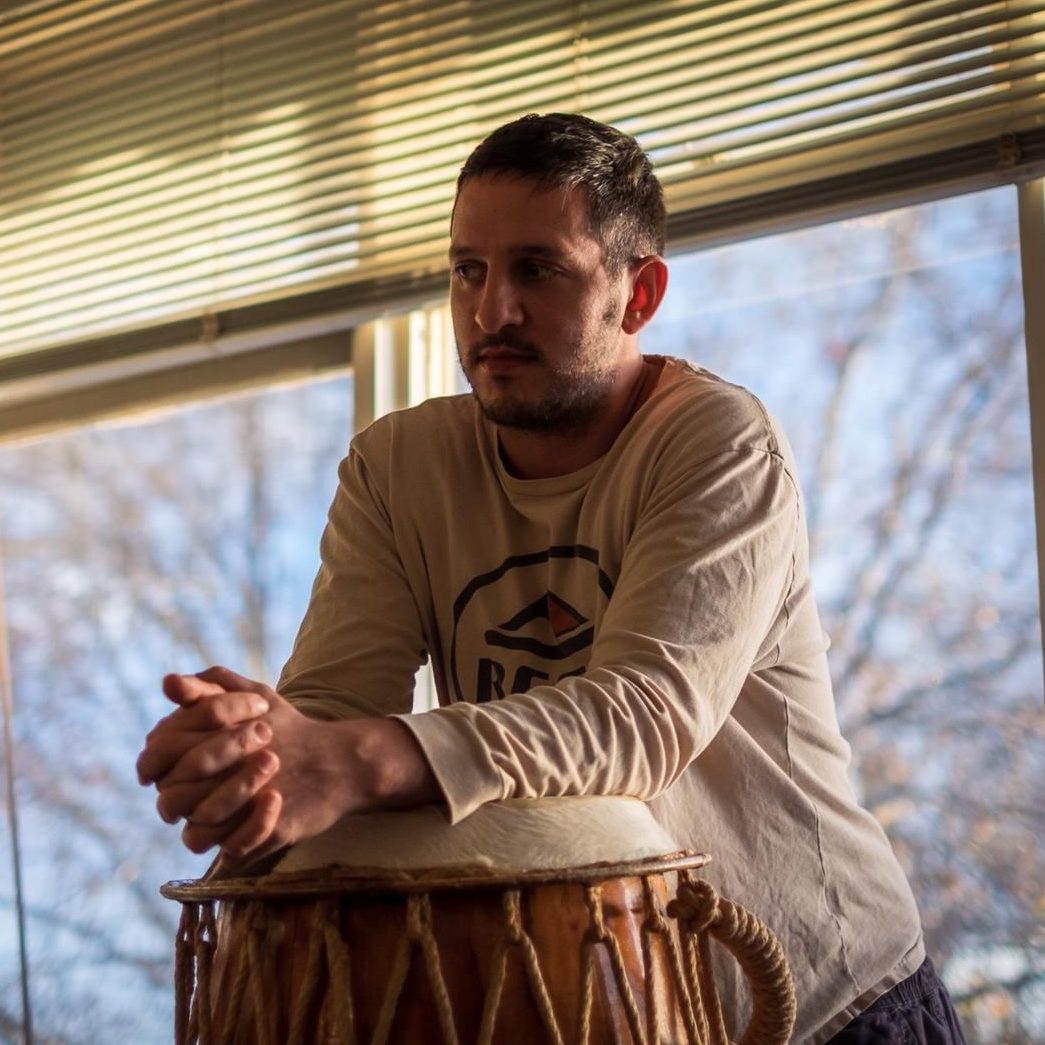


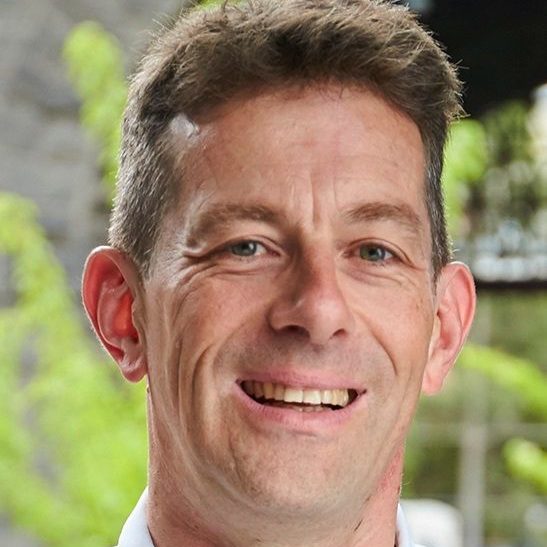

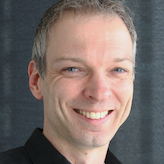
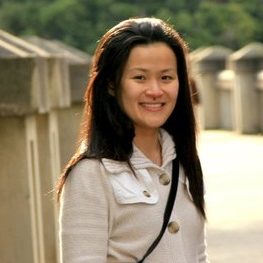

PARTNERS

Australian Broadcasting Corporation

Algorithm Watch (Germany)
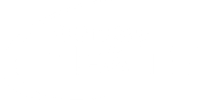
Bendigo Hospital

Google Australia
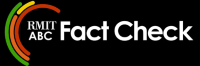